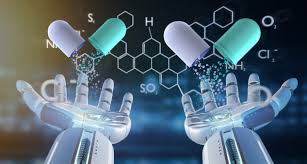
What is AI-Driven Drug Discovery?
AI-driven drug discovery is the application of artificial intelligence technologies to streamline and enhance the process of developing new medications. By utilizing AI algorithms, machine learning (ML), and deep learning (DL), researchers can accelerate the identification of promising drug candidates, predict their effectiveness, and optimize their development. This approach significantly reduces the traditional time and cost involved in the drug discovery process.
How Does It Work?
AI models are trained on large datasets containing biological, chemical, and medical information. These models can perform tasks such as:
- Molecule Design: AI algorithms can design novel molecular structures with the potential to target specific diseases, based on existing data on known compounds.
- Predicting Drug Interactions: AI systems can predict how different drugs will interact with the body and with each other, helping identify possible side effects and efficacy.
- Analyzing Clinical Data: AI tools are used to analyze vast amounts of clinical data to identify patterns, making it easier to predict patient responses to certain drugs.
- Automating Screening: AI can automate the high-throughput screening of chemical libraries, identifying the most promising compounds for further testing.
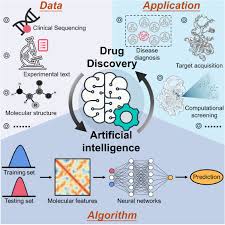
Key Technologies Involved
- Machine Learning (ML): Used to predict how molecules will behave and interact with disease targets, based on historical data.
- Natural Language Processing (NLP): Helps sift through and extract useful information from vast amounts of unstructured scientific literature and clinical trial reports.
- Generative Models: These models, like Generative Adversarial Networks (GANs), can create novel chemical compounds that might never have been considered by humans.
- Predictive Analytics: AI systems predict the likelihood of success in various phases of drug development, which helps prioritize research.
Benefits of AI in Drug Discovery
- Speed: AI can process and analyze data much faster than traditional methods, reducing the time from discovery to clinical trials.
- Cost Reduction: Traditional drug discovery can take up to a decade and cost billions of dollars. AI helps reduce these costs by streamlining processes and eliminating unnecessary steps.
- Targeted Treatments: AI models can help identify drugs tailored to specific patient groups, leading to more personalized and effective treatments.
- Drug Repurposing: AI can identify existing drugs that might be effective for new diseases, speeding up the process of finding treatments for emerging health crises.
- Predictive Accuracy: AI can predict the success rates of drug candidates before they undergo clinical trials, potentially preventing costly failures.
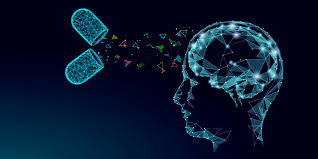
Challenges and Limitations
- Data Quality: The effectiveness of AI-driven drug discovery depends on the quality and quantity of data. Incomplete or biased datasets can lead to inaccurate predictions.
- Regulatory Hurdles: The FDA and other regulatory bodies need to adapt to AI-driven methods, which may take time, as they typically rely on traditional testing methods.
- Interpretability: AI models, especially deep learning algorithms, are often seen as “black boxes.” It can be difficult for researchers to understand exactly why a model made a particular prediction.
Current Progress and Companies Involved
Several companies and organizations are leading the charge in AI-driven drug discovery, including:
- Insilico Medicine: Known for using AI to design drugs targeting age-related diseases and other chronic conditions.
- Atomwise: Atomwise uses AI for drug discovery and has partnered with several organizations to discover new treatments for diseases like Ebola and multiple sclerosis.
- Exscientia: Focused on using AI to design small molecule drugs for various therapeutic areas, including oncology and immunology.
- BenevolentAI: This company applies AI to find new drug candidates for diseases like Alzheimer’s and Parkinson’s.
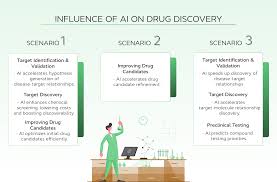
The Future of AI-Driven Drug Discovery
The integration of AI into the pharmaceutical industry is poised to reshape drug discovery. As AI models become more sophisticated and trained on larger, more diverse datasets, the accuracy and efficiency of the drug development process will continue to improve. This could lead to quicker responses to global health challenges, such as pandemics or antibiotic resistance, by significantly shortening the time it takes to develop effective treatments.
In the coming years, we may also see AI systems integrated with new technologies, such as blockchain (for secure data sharing), to further enhance collaboration and data transparency.
Conclusion
AI-driven drug discovery offers a promising future for the pharmaceutical industry, bringing us closer to faster, cheaper, and more personalized treatments. While challenges remain, ongoing advancements in AI and machine learning are likely to accelerate breakthroughs in healthcare.